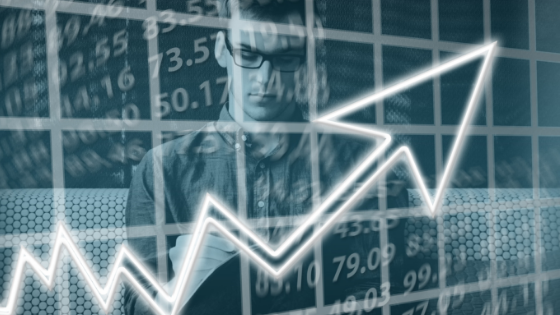
Things to consider when collecting sample data
If the sample data we collect is not collected appropriately, the data we gather may be completely useless.
An observational study allows us to measure certain characteristics, but we don’t modify the subjects which are being studied.
In an experiment we apply a treatment, then we observe its effects on a subject.
In an experiment, the group which is being treated will be compared to the group that did not receive any treatment. For example, let’s say the FDA chooses a random sample of aspirin in which they measure the accuracy. This would be considered an observational study since the FDA did not apply any treatment to the tablets but simply observing.
Different Types of Observational Studies
Cross-sectional study – in this type of study, the data is observed, then measured, and collected at one point in time. For example, a research company surveys about 5000 houses to determine who watches a certain tv show. They observe, measure, and collect these surveys, all at once.
Retrospective (also called case-control) study – our data values are collected from the past. This is done by going back into time (through records and examination, interviews, etc).
Then there is a prospective (or longitudinal or cohort) study. Here, data is collected in the future by groups that share common factors.
Issues to consider when collecting sample data
Control Effects of Variables – we should make sure not to let other variables interfere with any. effects we want to see.
Replication and Sample size – the sample size should be large enough so we are able to see the effects and their true nature. Making sure we use an appropriate method is important, such as one that is based on randomness.
Following the above considerations, we can confidently use data, not only because of sample size but also when replicating the study in the future.
What is Randomization?
Randomization – using a random procedure to collect individual sample items.
In a random sample, those in a population are selected in a way that gives each individual member an equal chance of being selected.
There are many ways to collect a random sample. The important thing is making sure each member has an equal chance of being selected.
Then there is a simple random sample. A simple random sample of size n subjects is chosen in a way that every possible data sample of the same size n has an equal chance of being chosen.
A probability sample selects members from a population in such a way that each member is known but not necessarily the same chance of being selected.
Example of Probability Sample, Simple Random Sample, and Random Sample
Conceptualize a classroom with 60 students who are arranged in 6 rows of 10 students each. Pretend the professor selected a sample of 10 students by the roll of a die, then selecting the row which corresponded to the outcome. Because all the students have the same chance of being selected, this would be an example of a random sample. Every student has a 1:6 chance of being selected. It is not a simple random sample since the sampling design (using a die) does not allow us to select 10 students who are in different rows, so we can’t have different sets of 10, only the particular rows. But it is a probability sample since each student has a known chance (1/6) of being selected.
Other Kinds of Sampling
Systematic sampling – one selects a starting point and then must select kth (for example ever 5th) element in a population. For example, from our starting point, we would select every 5th element. That would make up our sample.
With convenience sampling, we simply utilize results that are easy to get. (Choose the sample that is easiest to get).
Stratified sampling – when one subdivides population into at the minimum, 2 groups. These groups share the same characteristics. This can be age, gender, hair color, etc. Then we get a sample from each subgroup (also called stratum). So if we chose the data, age, we then draw a sample from those subgroups.
In cluster sampling, one divides the population into sections (also called clusters), then we randomly select some of the clusters, and then we choose the members from those clusters.
For example, if a policeman would stop and interview every 5th driver, that would be systematic sampling. Where k is 5 since every 5th driver was selected.
If a program was to randomly select 5 different classes and interviewed all students in those classes that would be an example of cluster sampling.
Conclusion, 2 types of errors to watch out for
Sampling error – this is the difference between a sample result and the actual population result. This error results from a chance fluctuation of the sample.
Nonsampling error – this occurs when sample data is not correctly collected, analyzed, or recorded (such as using a biased sample or defective instrument, or not copying the data correctly).